João Carvalho
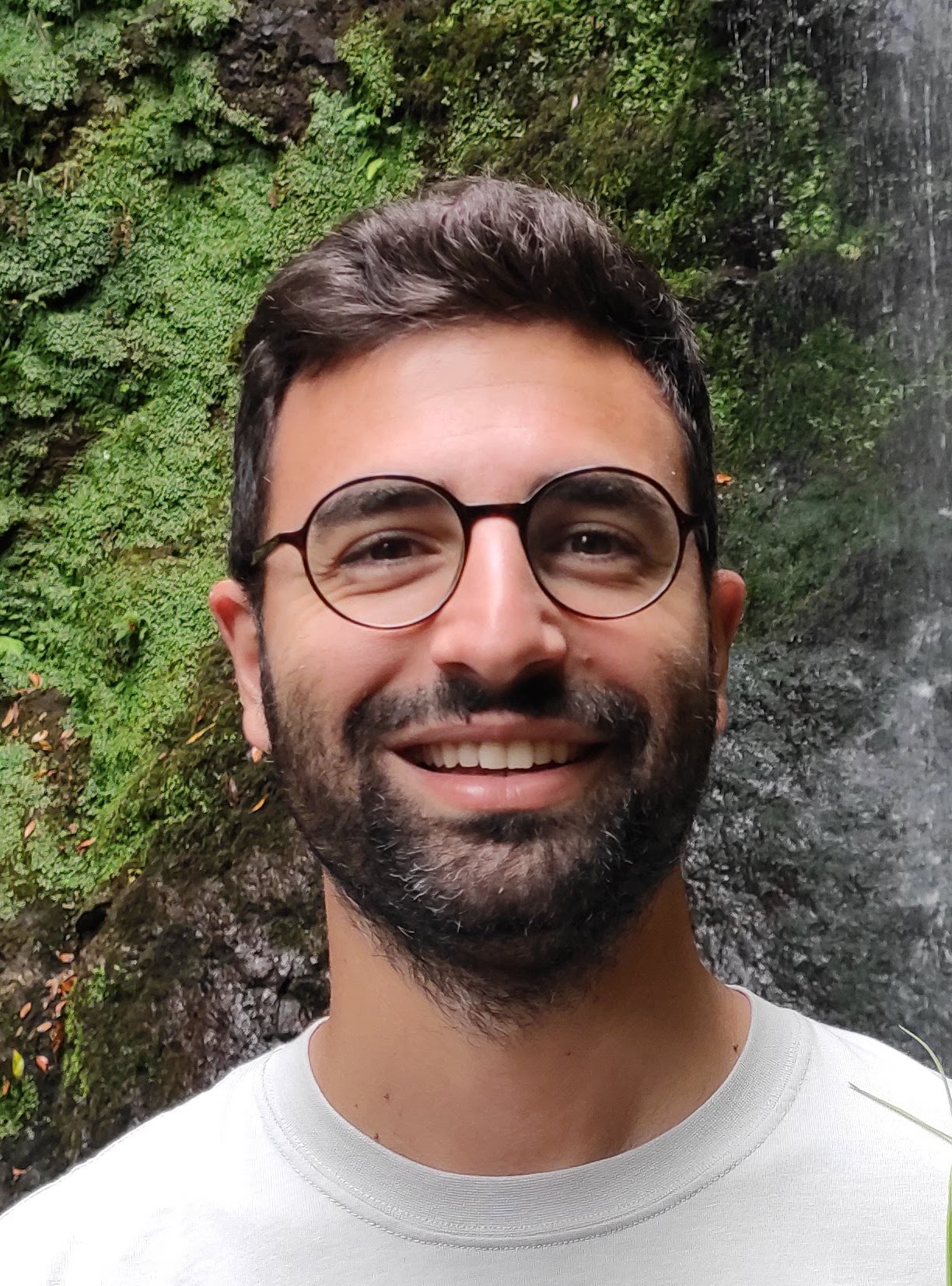
Research Interests
- Robotic Manipulation
- Learning Motion Planning
- Reinforcement Learning for Robotics
Affiliation
TU Darmstadt, Intelligent Autonomous Systems, Computer Science Department
Contact
joao.correia_carvalho@tu-darmstadt.de
joao@robot-learning.de
Room E325, Building S2|02, TU Darmstadt, FB-Informatik, FG-IAS, Hochschulstr. 10, 64289 Darmstadt
+49-6151-16-25372
João joined the Intelligent Autonomous Systems group as a Ph.D. student in November 2019. He received a M.Sc. degree in Computer Science from the Albert-Ludwigs-Universität Freiburg, and previously completed a Master's degree in Electrical and Computer Engineering from the Instituto Superior Técnico of the University of Lisbon. His master thesis was written at IAS under the supervision of Samuele Tosatto and explored an approach to obtain an off-policy gradient with higher sample efficiency.
Currently, he is working within the IKIDA project to develop algorithms that enable robots to work alongside humans.
During his Ph.D. João is developing learning algorithms for robotic manipulation. These include methods that leverage generative models for motion planning, reinforcement learning methods to solve contact-rich tasks like insertions, or improving policy gradient methods with variance reduction techniques.
Students looking for Master Thesis: I'm offering one topic in Geometry-Aware Diffusion Models for Robotics (please check https://www.ias.informatik.tu-darmstadt.de/Theses/OpenTopics, and contact me if you are interested).
Publications
Motion Planning with Diffusion Models
-
- Carvalho, J.; Le, A. T.; Baierl, M.; Koert, D.; Peters, J. (2023). Motion Planning Diffusion: Learning and Planning of Robot Motions with Diffusion Models, IEEE/RSJ International Conference on Intelligent Robots and Systems (IROS).
-
- Carvalho, J.; Baierl, M; Urain, J; Peters, J. (2022). Conditioned Score-Based Models for Learning Collision-Free Trajectory Generation, NeurIPS 2022 Workshop on Score-Based Methods.
Reactive Motion Planning
-
- Vorndamme, J.; Carvalho, J.; Laha, R.; Koert, D.; Figueredo, L.; Peters, J.; Haddadin, S. (2022). Integrated Bi-Manual Motion Generation and Control shaped for Probabilistic Movement Primitives, 2022 IEEE-RAS 21st International Conference on Humanoid Robots (Humanoids).
Robot Learning for Contact-Rich Manipulation
-
- Carvalho, J.; Koert, D.; Daniv, M.; Peters, J. (2022). Adapting Object-Centric Probabilistic Movement Primitives with Residual Reinforcement Learning, 2022 IEEE-RAS 21st International Conference on Humanoid Robots (Humanoids).
Reinforcement Learning and Policy Gradients
-
- Palenicek, D.; Lutter, M.; Carvalho, J.; Peters, J. (2023). Diminishing Return of Value Expansion Methods in Model-Based Reinforcement Learning, International Conference on Learning Representations (ICLR).
-
- Carvalho, J.; Peters, J. (2022). An Analysis of Measure-Valued Derivatives for Policy Gradients, Multi-disciplinary Conference on Reinforcement Learning and Decision Making (RLDM).
-
- Carvalho, J., Tateo, D., Muratore, F., Peters, J. (2021). An Empirical Analysis of Measure-Valued Derivatives for Policy Gradients, International Joint Conference on Neural Networks (IJCNN).
-
- Tosatto, S.; Carvalho, J.; Peters, J. (2022). Batch Reinforcement Learning with a Nonparametric Off-Policy Policy Gradient, IEEE Transactions on Pattern Analysis and Machine Intelligence (PAMI), 44, 10, pp.5996--6010.
-
- Tosatto, S.; Carvalho, J.; Abdulsamad, H.; Peters, J. (2020). A Nonparametric Off-Policy Policy Gradient, Proceedings of the 23rd International Conference on Artificial Intelligence and Statistics (AISTATS).
-
- Carvalho, J.A.C. (2019). Nonparametric Off-Policy Policy Gradient, Master Thesis.
Supervised Theses and Projects
Teaching Assistant
Lecture | Years |
Computational Engineering and Robotics | SS 2020, SS 2021 |
Robot Learning | WS 2020 |
Robot Learning Integrated Project | SS 2022 |